Nonrigid Image Matching Benchmark
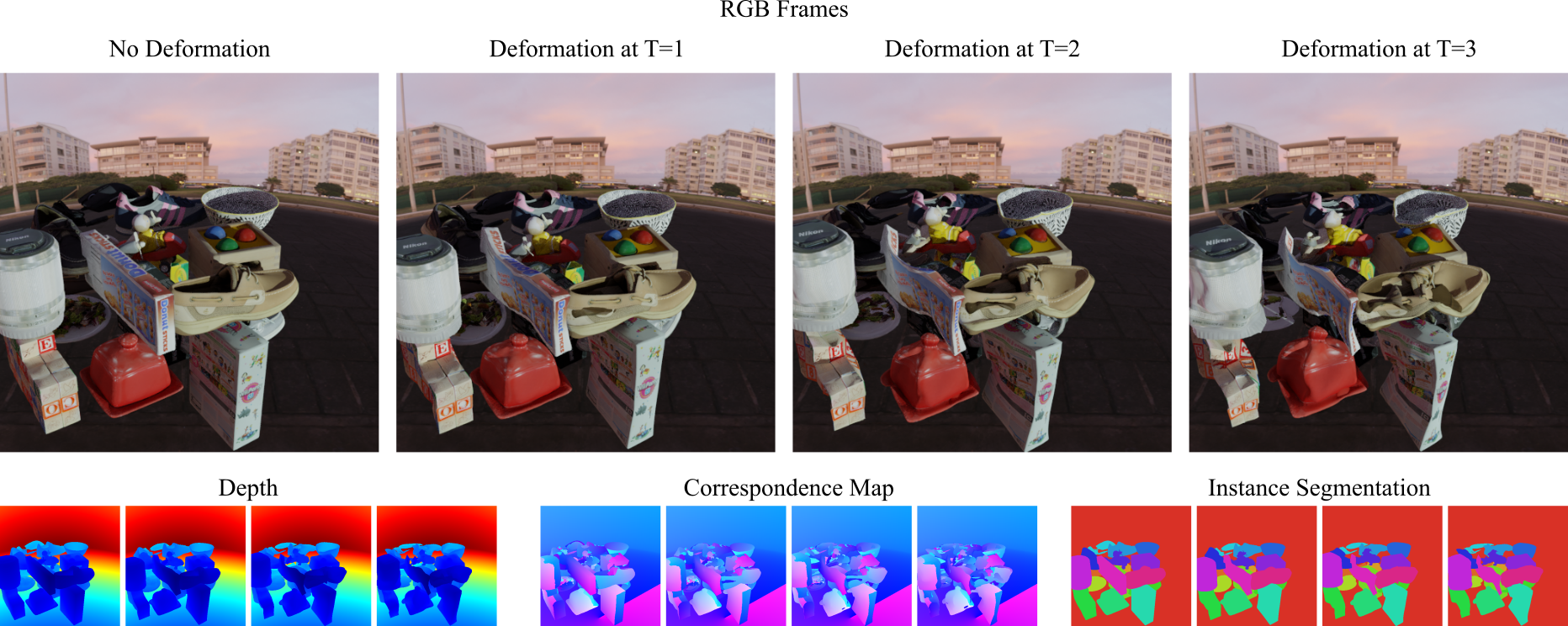
Gathering accurate and diverse data for nonrigid visual correspondence poses a significant challenge because of the time-consuming annotation process and the inherent difficulty in manually controlling surface deformations. The scarcity of high-quality visual data with deformations hinders the evaluation and training of visual correspondence methods. To overcome these problems, we introduce a novel photorealistic simulated dataset designed for training and evaluating image matching methods in nonrigid scenes. The proposed dataset contains temporally consistent deformations on real scanned objects, ensuring both photorealism and semantic diversity. Unlike existing datasets, our approach enables the extraction of accurate and dense multimodal ground truth annotations while maintaining full control over the scenarios and object deformations. Our experiments demonstrate that current nonrigid methods still have room for improvement in deformation invariance, highlighting the importance of data containing representative deformations. In addition to releasing a training dataset with 2M pairs (including 830 unique objects with 4 levels of deformations) and fine-tuned weights for popular learned-based feature description methods, we also provide a private evaluation set with 6000 pairs through an online benchmarking system to promote a standard and reproducible evaluation for future works.
Visualizing Matches Across Deformations
Try to change the transformations!
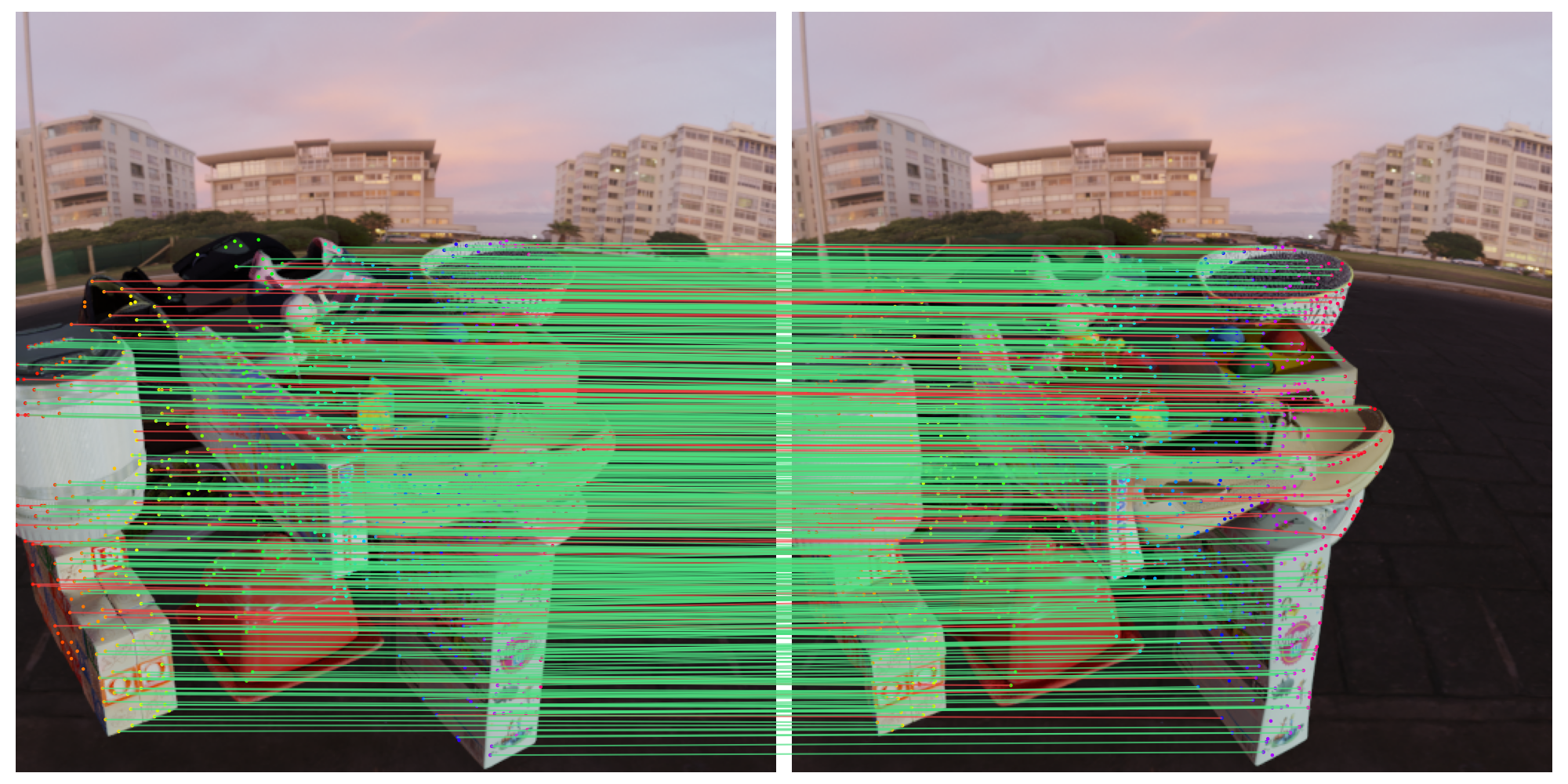
Low
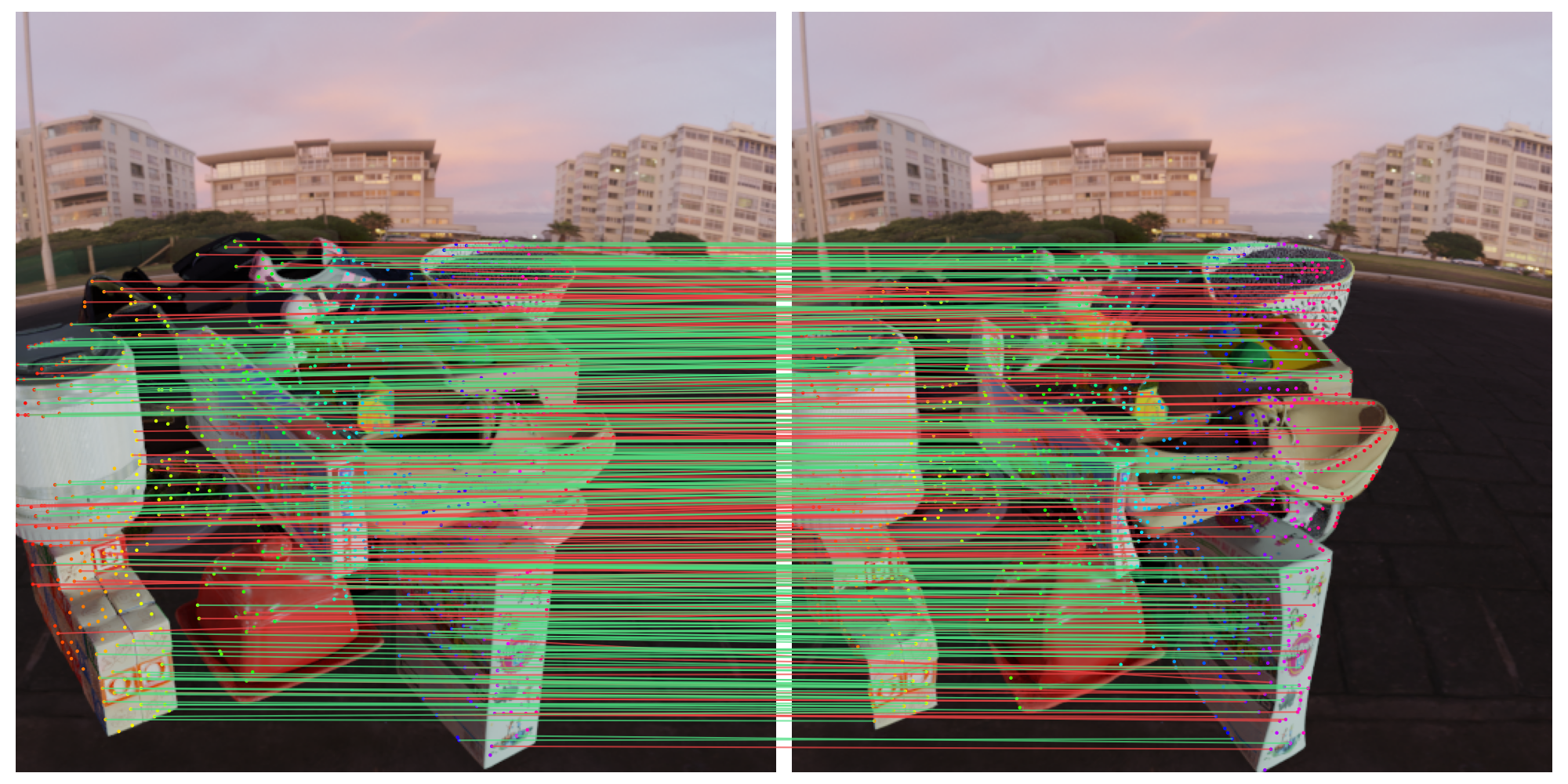
Medium
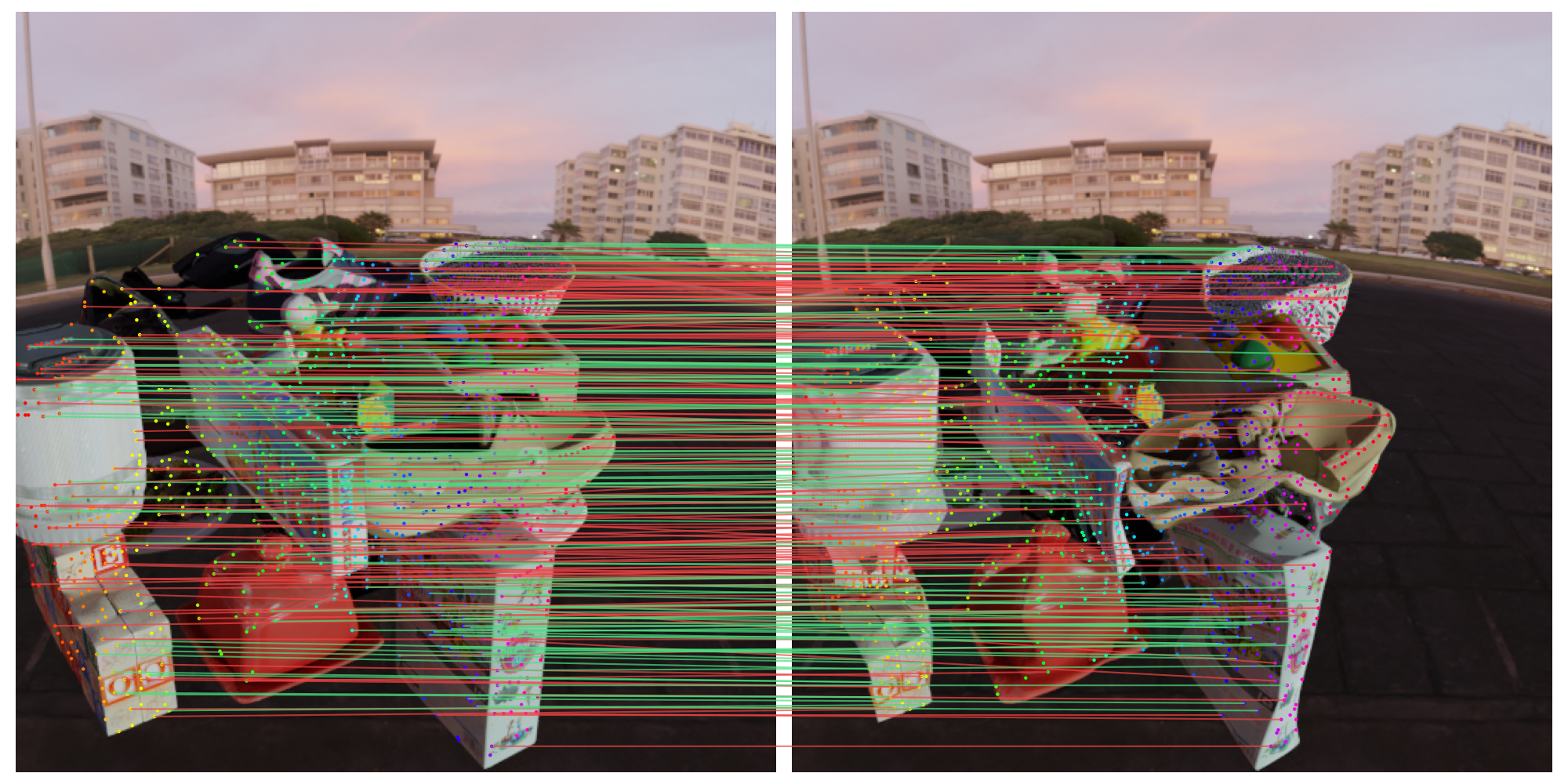
High
Deformation Examples
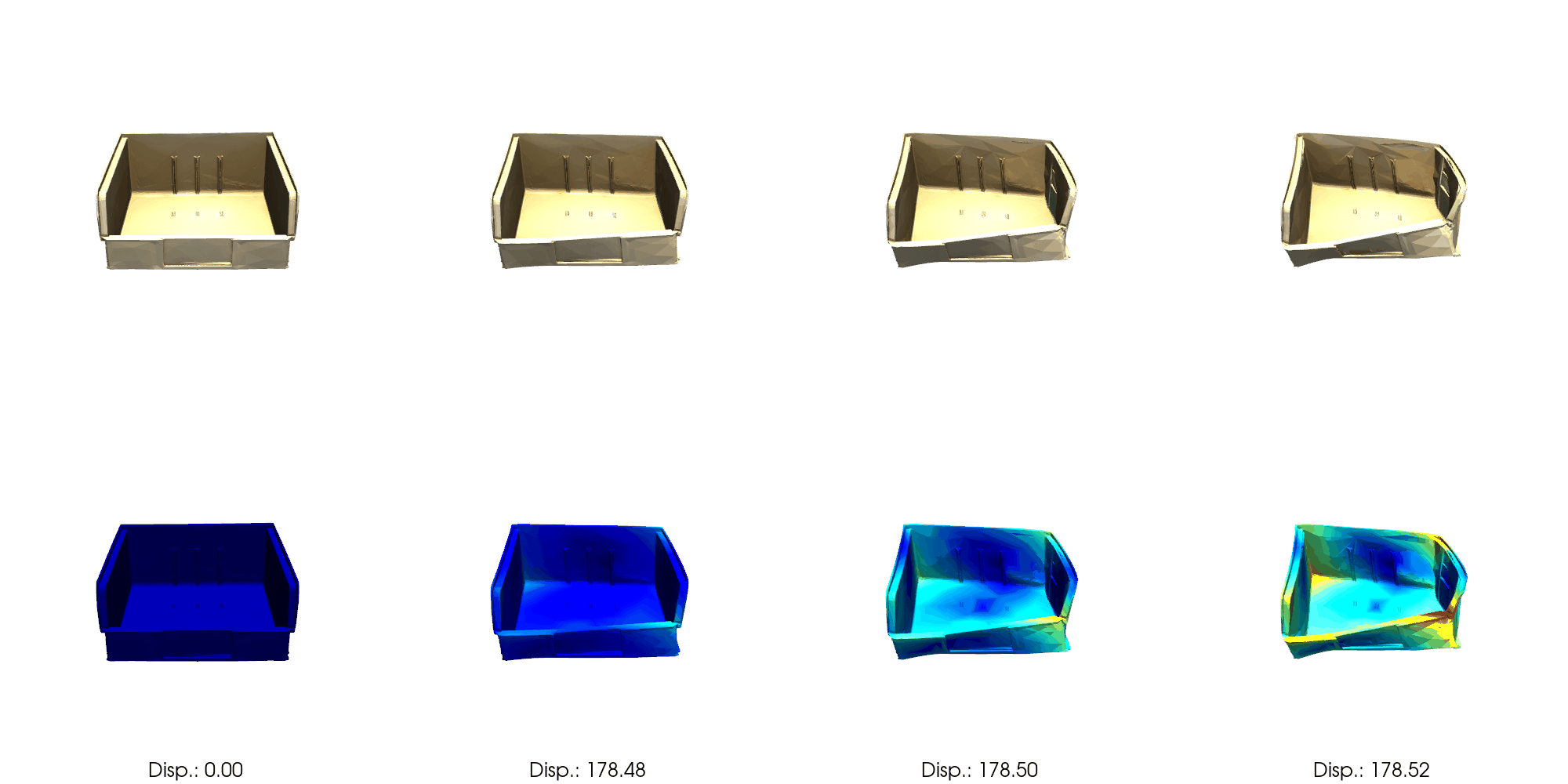
Deformation Example 1